White Paper: Theia Markerless motion capture validation
​
Synopsis: Theia Markerless motion capture uses video cameras and deep-learning algorithms to measure human motion. This white paper summarizes three peer-reviewed articles that assess the accuracy and repeatability of this markerless system. In all experiments, the markerless system performed comparably to the gold standard, validating its use as a measurement system for human movement.
Prelude:
One of Theia’s founding principles is integrity, and it resonates throughout our ecosystem. Our customers require data integrity, and we believe it's our responsibility to provide an impartial assessment of our software to demonstrate that it meets the high standards of the research community. To perform this assessment we collaborated with HMRL at Queen’s University (supervised by Dr. Kevin Deluzio). The objective was to compare the measurements made with the Theia Markerless system to those made with a marker-based system widely used in the research community and commonly accepted as the gold standard.
Our contributions to the project were to assist in the experimental design, provide funding for equipment and personnel, and provide software for analysis. We did not perform any of the analysis, or have any day-to-day input to the project. Our approach was to remain impartial. This collaboration resulted in three peer-reviewed research articles, which are summarized in this white paper.
The purpose of this paper is not to reiterate the rigorous analysis described in the articles - if you would like more details on the methods and results of these studies, please read the articles (references are provided at the end of this document). Rather, the purpose is to provide a snapshot of the research and some general thoughts on the capabilities of our markerless system. Because we believe that system assessment is an on-going process, this white paper will be a living document that is modified whenever new information is available. Currently, there are ~15 additional assessment studies in progress.
Paper 1: Assessment of spatiotemporal gait parameters using a deep-learning algorithm-based markerless motion capture system
Rational: The first study examined basic spatial and temporal walking parameters, such as walking speed, stride width, and stride length. These parameters, which are commonly used to characterize and evaluate walking, are typically good indicators of health and function, and are fairly simple to collect and analyze. Investigating these parameters was a natural first step in assessing the performance of the system; if it does not perform well on these measurements there is no point in continuing on to study full-body joint kinematics.
Methods: Study participants were measured during normal walking in a lab setting for two experiments: the first comparing marker-based motion capture (Qualisys) to markerless motion capture, and the second comparing a pressure mat (GaitRite) to markerless motion capture. For each experiment, a roughly even male/female ratio was collected, with 30 and 25 participants respectively. Using these walking data, a typical set of spatial temporal parameters were calculated (Table 1a).
Results:
Table 1a: Summary of mean differences for spatial temporal parameters
​
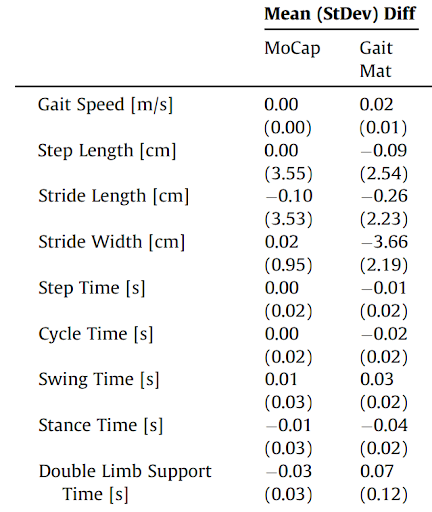
Discussion: The statistical analysis of the distance-based metrics showed good to excellent agreement between the markerless motion capture and markered/pressure mat systems. The one exception was stride width, where pressure mat data showed differences from both the marker-based and markerless systems. This was likely a result of low resolution in the width dimension of the pressure mat system.
The time-based metrics showed a slightly lower agreement. This was partially attributed to inaccuracy in event detection, as these were determined using kinematic events and not force plates. Kinematic events are accurate to +/- 1 frame, and the mean errors measured (relative to GaitRite) were between 0-4 frames (markerless) and 0-3 frames (marker-based) for the experiments. So while there was slightly lower agreement, the markerless and marker-based systems performed similarly. For some perspective, if you were to review video and scroll through frames one by one, it would be difficult to determine these gait events to this degree of accuracy.
Given that the markerless system measured effectively the same values as both the marker-based system and the pressure mat, the authors deemed that the markerless system was sufficiently accurate for this type of measurement.
Paper 2: Concurrent assessment of gait kinematics using marker-based and markerless motion capture
Rational: Marker-based motion capture is the most common modality for measuring human motion, and although there are few validation studies for its accuracy, it is widely accepted as the gold standard in movement measurement. As a result, comparison to marker-based motion capture was a natural progression to assess the capabilities of Theia’s markerless system.
Methods: 30 healthy, young individuals were collected while walking on a treadmill. The measurement system used was a combination of 8 video cameras, and 10 infrared cameras (Qualisys), that was capable of concurrent recording (fully synchronized) using the same global calibration frame for all cameras. The data from both systems were examined from heel strike to heel strike, comparing joint angles, segment angles and joint positions.
Results: Joint positions between the collections were within 2.5 cm for all joints, other than the hip, where they were within 3.5 cm. Lower limb segment angles were within 5.5 degrees, other than those rotating about the long axis of the segments, which were slightly larger.

Figure 1b: Segment angles from heel strike to heel strike, for all signals, for all strides
Discussion: The differences between the markerless and marker-based measurements were less than the typical differences between two marker-based systems that use different marker sets.
The primary and secondary segment angles (the segment angles other than the long axis rotation) were very similar for both systems (differences ~2 degrees). The differences here are well within the range of segment angle differences associated with repeated marker placements presented in the literature. Tertiary joint angles (along the long axis of segments) differed by more. However, soft-tissue artefact and marker placement affect these angles significantly, making it difficult to assess which system is more accurate. In any case, the differences measured between the markerless and marker-based systems are less than can be perceived by eye, making it difficult to distinguish between the two when visually examining the animated skeletons (Fig. 2b).
This study provided a great baseline for comparison. With additional resources we would also like to:
-
Compare to other marker sets, to better understand their effect on the joint angles
-
Remove and re-apply the markers, then repeat the measurements
-
Have a second experimenter collect the same data
-
Repeat the experiment on a different day
Although some of these issues are addressed in the subsequent experiment, this comprehensive set of experiments would provide more insight into the differences between the systems and the variability inherent in motion-capture measurements (i.e., independent of the capture technique).
***A very important note is that the Theia pose-estimation algorithms have never been trained on marker data or images of the lab where this experiment took place. Adding marker-based data and context-specific data from this lab would likely further improve the accuracy, however that would not necessarily translate to other settings. This is why we elected not to add data from this environment, so the results better reflect what a user would experience. We highly question any validation work that does not follow this underlying principle. Secondly, because the algorithms have not seen marker data, it’s very likely that their presence has actually made the results presented here worse than if we had added markers to the training set. However, since our typical user does not apply markers to the subject, we have again elected not to train on a data set with markers, because the results and their accuracy may not transfer to a typical user. Again, we highly question any “validation” that does not adhere to this strict sampling of data.***
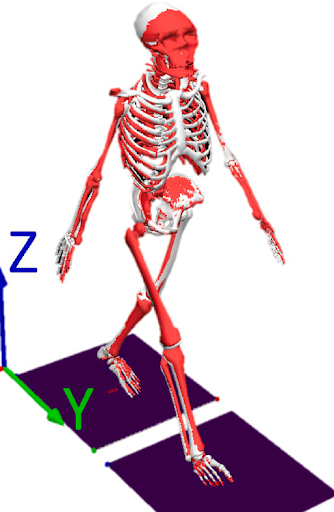
Figure 2b: Cool overlay, showing marker-based skeleton in red, and markerless skeleton in white
Paper 3: Inter-session repeatability of markerless motion-capture gait kinematics
Rational: After demonstrating that the accuracy of our markerless system is comparable to the gold-standard marker-based system, the next step was to evaluate its repeatability. Repeatability is very important for measurement systems, because it allows for experiments to be replicated consistently, which is an essential characteristic of a good experiment. Furthermore, any study that measures transient effects, such as an intervention study, requires high repeatability (in order to accurately quantify the effects of the intervention). Regardless of accuracy, if nothing changes with the movement, it is crucial that a system produce the same measurements on different days.
Methods: Nine participants were recorded with the markerless system on three separate days performing over-ground walking trials. These participants were not instructed to wear the same clothing everyday, but to come wearing “normal” clothing (Figure 1c). Inter-trial and inter-session variability were examined for lower-body joint angles for all participants. Inter-trial variability is a measure of intrinsic differences in walking patterns from stride to stride, as well as the system noise. Inter-session variability is a measure of how much variation is introduced by the experimental setup (clothing, lighting conditions, subject mood, etc.).
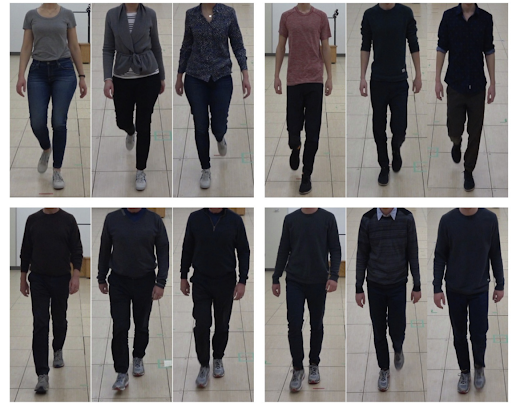
Figure 1c: Sample clothing for four participants, on three different days.
Results: Mean joint angles were consistent between collections (Figure 2c). Inter-trial variability was slightly higher than previously reported studies, averaging 2.5 degrees, while inter-session variability was considerably smaller, at 2.8 degrees. Effectively, the multi-session protocol increases the variability of the data collection by 10%.
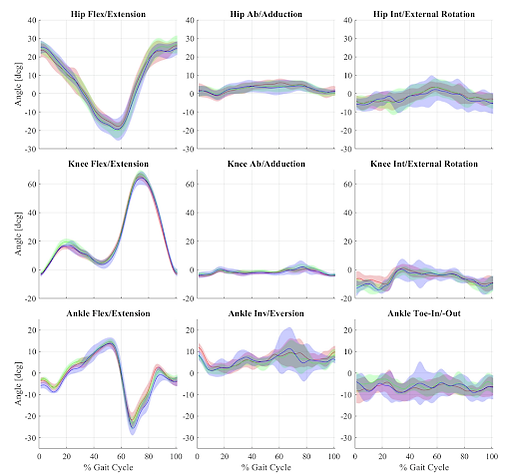
Discussion: Typically, multi-session protocols collected using marker-based motion capture can introduce anywhere between 2 and 5 degrees of variation in joint angles. In our experience, this variability is more likely at the higher end of the range, and can be far worse with inexperienced investigators. While the markerless system showed slightly higher variability within trials, it had a smaller variability between sessions than marker-based collections.
The mean joint angles between the three collections were statistically the same. This is an important outcome because clothing was not controlled, indicating that not only is the system not sensitive to the day-to-day changes, but the markerless system is not affected greatly by clothing choice either (to a degree, assuming the clothing follows the recommendations). This reinforces general robustness, and allows participants to avoid wearing uncomfortable and sometimes demeaning lab clothing. We believe that if each subject wore the same clothing in all three sessions, the results would be more similar.
An important benefit of a system that is not dependent on the experimenter is that it enables labs to collaborate by sharing data. Often, there are varying levels of skill between sites that wish to collaborate, and pooling data is generally challenging. Differences in marker set, marker placement, and lab expertise and protocol are all avoided with robust algorithms. In the future, we hope to repeat the inter-session experiment but measure the same set of subjects at different labs, to better demonstrate the benefits of markerless capture for collaboration between labs.
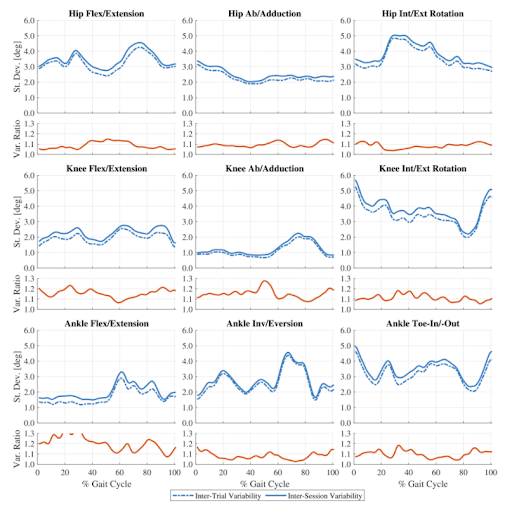
References: